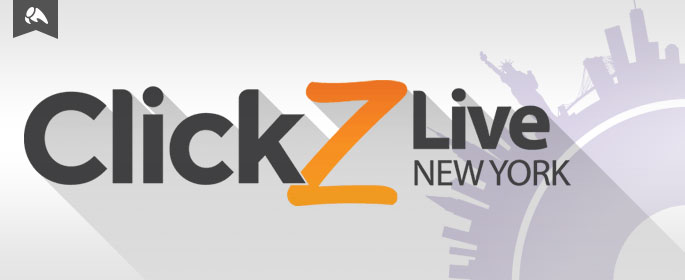
ClickZ New York’s opening keynote started the morning off well. Now, my pick for sessions today is “Ultimate Engagement: The Power of Data-Driven Storytelling,” with Dr. Jon Roberts of About.com. With a PhD in theoretical high energy physics and previous studies in dark matter, I’m pretty intrigued to hear about his transition to being the director of data sciences for About.com’s content. The first question he had to answer at About.com – “Why did we hire a dark matter physicist?”
Physics is all about predicting the future from messy, time series data. Science is the process of extracting meaning from data.
“Understanding seasonality in a detector in the Andes is the same as understanding seasonality of “when is Easter” searches.”
When you look at data, it’s important to know what the data is telling you. If you can’t tell a story at the end of your data, that data wasn’t valuable in the first place.
How do scientists tell stories with data?
- Identify the question.
- Pin down your intuitions – think about what you think the answer is going to be.
- Get the (right) data.
- Analyze!
- Tell the story.
- Extra credit: Build the tool that lets others tell their own story.
Identify the Question
There is a lot of data out there. But very few of those things you can find out are worth knowing. It’s not worth looking at data for the sake of looking at data. “You can disappear for days and not come back with anything useful.”
So identify the question you really want the answer to. Can data help you prove someone wrong (especially yourself)? Can you make a decision once you’ve answered your question?
First question tackled at About was “What is About about?”
Identify Your Intuitions
This is a key step before analyzing the data. You should remember what you thought before you did your analysis. You should have some idea of what a reasonable result of data analysis may be.
When you find out you were wrong, you need to remember so that you can explain that part carefully. Remember where you started from, because whoever you have to communicate these results to are probably starting with the assumptions you had, as well.
We already know what a “reasonable” rent is, in New York or Detroit. We know a reasonable cost for a latte. Your intuitions are data-driven. We’ve acquired this knowledge over time.
What this means is that when you see a $20 latte, you know there’s something weird going on. The exact same thing is true when you do data analysis. You should have expectations.
Intuitions: What is About about? About is older than Google. About has been a top 20 site for almost all of the last 18 years. About is evergreen reference content. About isn’t very time dependent.
Get the Right Data
You don’t need big data for data science. Your data might come from big data, but most analyses use data that could fit on a USB stick. All big data storage is built to quickly extract just the snapshot of the data you need to answer the question. Find the small data set you actually need.
What is big data?
- All monthly page views for all sites on About back to 2000. 157 rows, 2000 columns, 1.8Mb. Not big data. You can email that to someone.
- War and Peace. 587,287 words, 7.2Mb. Not big data.
- All 311 calls in 2009. 1.8m rows, 875 Mb. Not big data.
- All click data on About – approx 100Tb. That’s probably big data.
- Large Hadron Collider results – 25 petabytes a year. That’s huge data.
But which one of those data sizes is actually going to be helpful to you? How much do you need to analyze your marketing? Probably not “big” data. A small excerpt.
Analyze the Data
Find all the things that are weird. Look at the minimum, the maximum, the zeros, the missing data, the outliers. First, find the things that are wrong with your data. Things happen. Files get corrupted. Errors are made. Find the things that shouldn’t be what they are.
Then, create a ton of plots. What are the storylines that could create this data?
Next, find where your intuitions are wrong. What has your data taught you that you didn’t assume? Is it you, or is it the data? If it’s the data, clean that data up.
Tell a Story
What did I think when I started? Why was that wrong? What do I think now and why?
Take those answers and illustrate the story with a few key plots. Tell how you got to where you are, pointing out all the places you were surprised.
A few About stories:
About found that Internet users are largely interested in the same topics year after year (growing, as use of the Internet rose). Careers advice. Home advice. These needs and questions haven’t changed tremendously. About found year after year its traffic slows in December (“people are with their families, so they’re asking their families questions instead of the Internet.” Go figure).
About can also identify data that correlates (spike in military topics corresponds with spike in humor views – people need a laugh). Low carb diets has gotten more interest overtime. Weight loss and Islam have a spike in September 2001 – the only two parts that had significant spikes in that month. After 9/11, did people, faced with their own mortality, decide they were going to get healthier?
About can also see “cellphones” site lose out to iPhones and smartphones, over time. But their combined interest remains the same over time.
Wrap-up
Data can be tremendously helpful in understanding your audience’s interest and knowing what content you need to publish moving forward. Understand what data is relevant (this may take some exploration), learn from it, communicate your learnings to the rest of the team, and then act on it.
And remember, machine-learning can’t replace human learning. Make sure the data’s results makes sense. Algorithms aren’t going to tell you everything.